Smart Homes Research
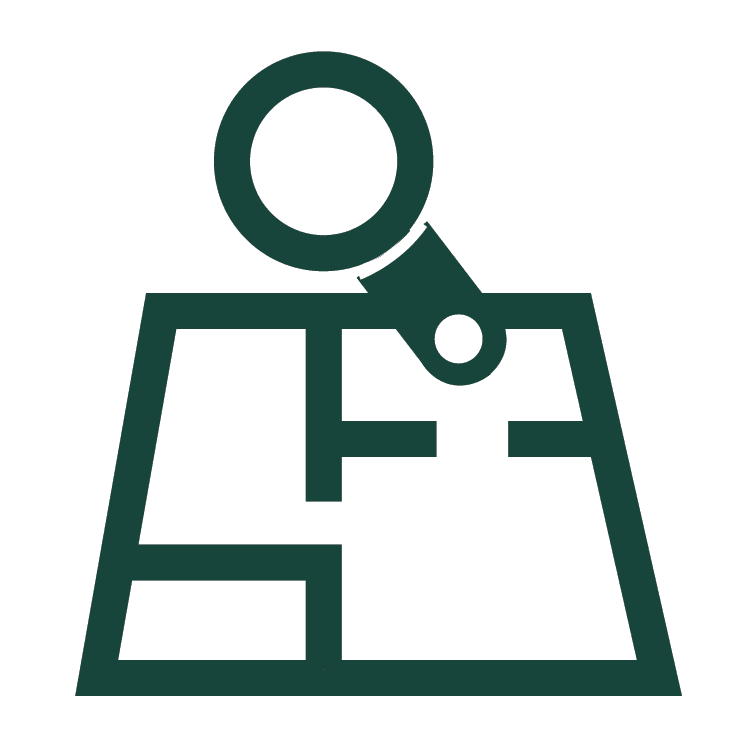
Occupant Detection & Indoor Mapping
Technologies developed by MSU Faculty help Smart Homes map the interior of a space allowing systems to be custom tailored and safer.
- Self-improving indoor localization by profiling outdoor movement on smartphones
- iFrame: Dynamic indoor map construction through automatic mobile sensing
- Cooperation among smartphones to improve indoor position information
- EyeLoc: Smartphone Vision Enabled Plug-n-play Indoor Localization in Large Shopping Malls
- Typical occupancy profiles and-behaviors in residential buildings in the United States
- NestDNN: Resource-aware multi-tenant on-device deep learning for continuous mobile vision
- Interaction effects of building technology and resident behavior on energy consumption in residential buildings
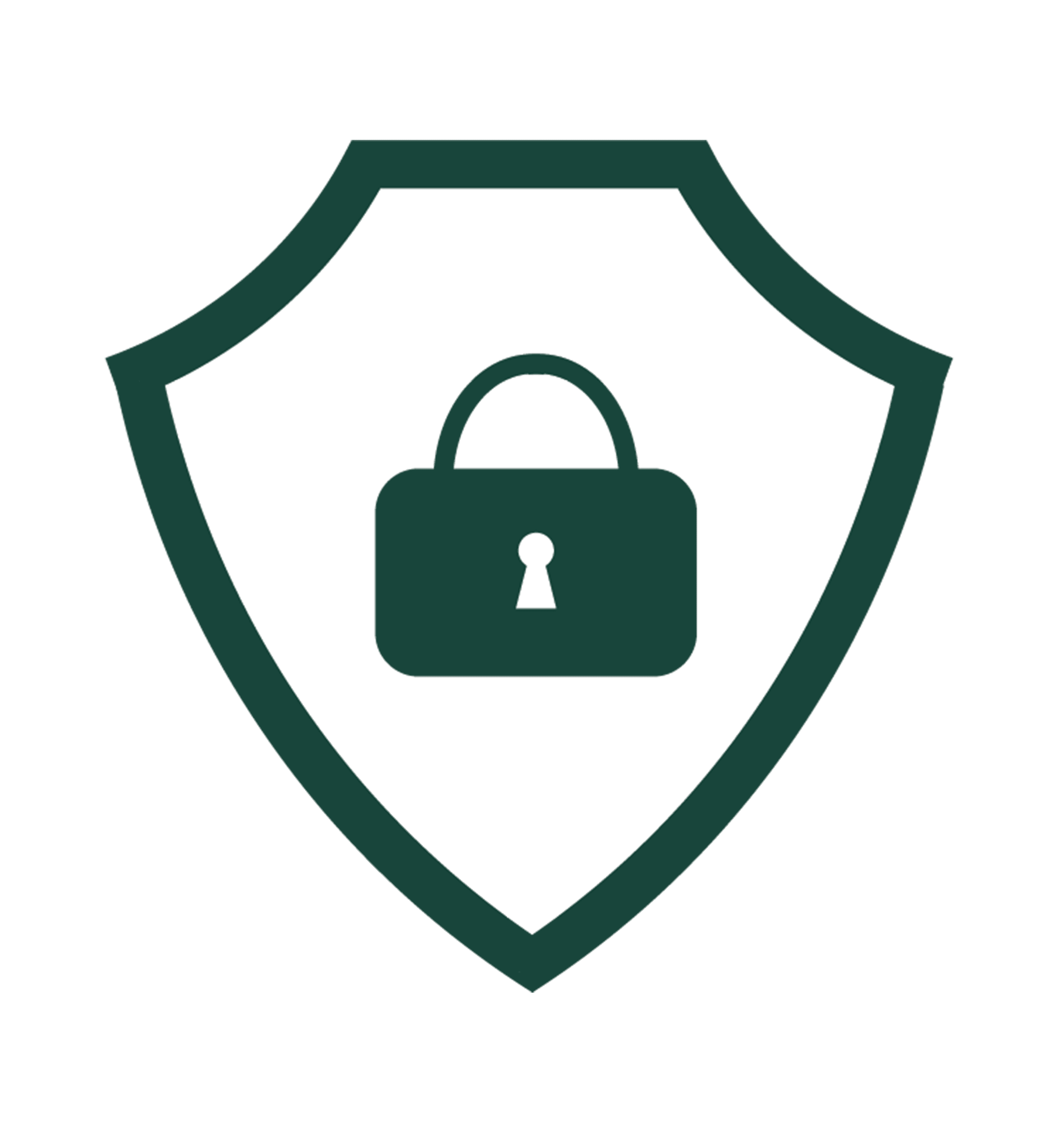
Smart Home Cyber Security
- SecWIR: securing smart home IoT communications via wi-fi routers with embedded intelligence
- Access control with delegation for smart home applications
- Secure wireless monitoring and control systems for smart grid and smart home
- RELAX: a language to address uncertainty in self-adaptive systems requirement
- A Lightweight Block Validation Method for Resource-Constrained IoT Devices in Blockchain-Based Applications
- CapChain: A Privacy Preserving Access Control Framework Based on Blockchain for Pervasive Environments
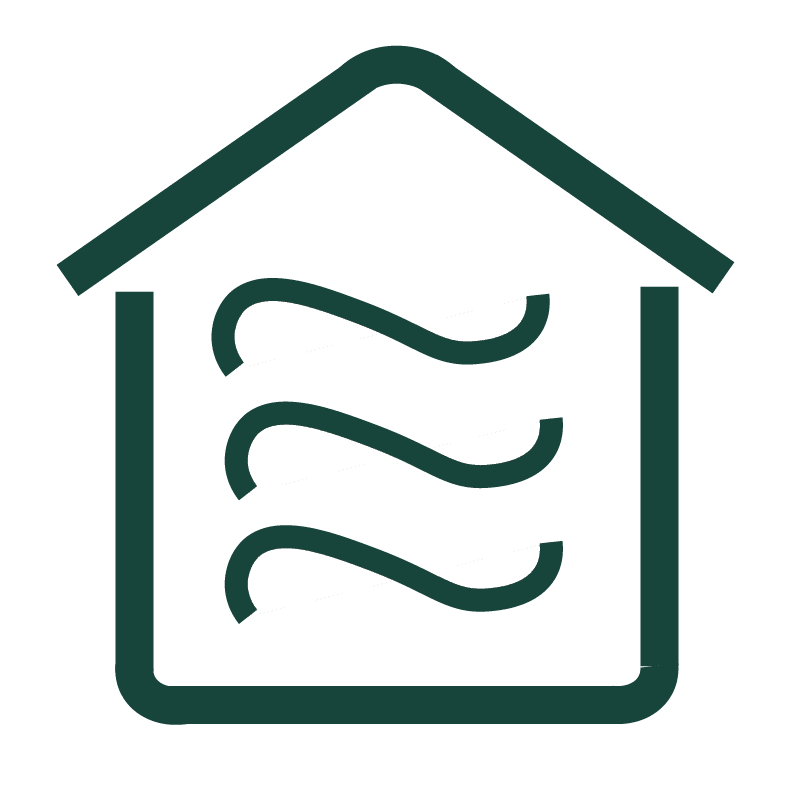
Indoor Air Quality
Poor indoor air quality can have a number of negative health consequences if left undiscovered. Researchers at MSU are working to enhance the capabilities for consumers to monitor the air quality within their homes using connected air quality sensors.
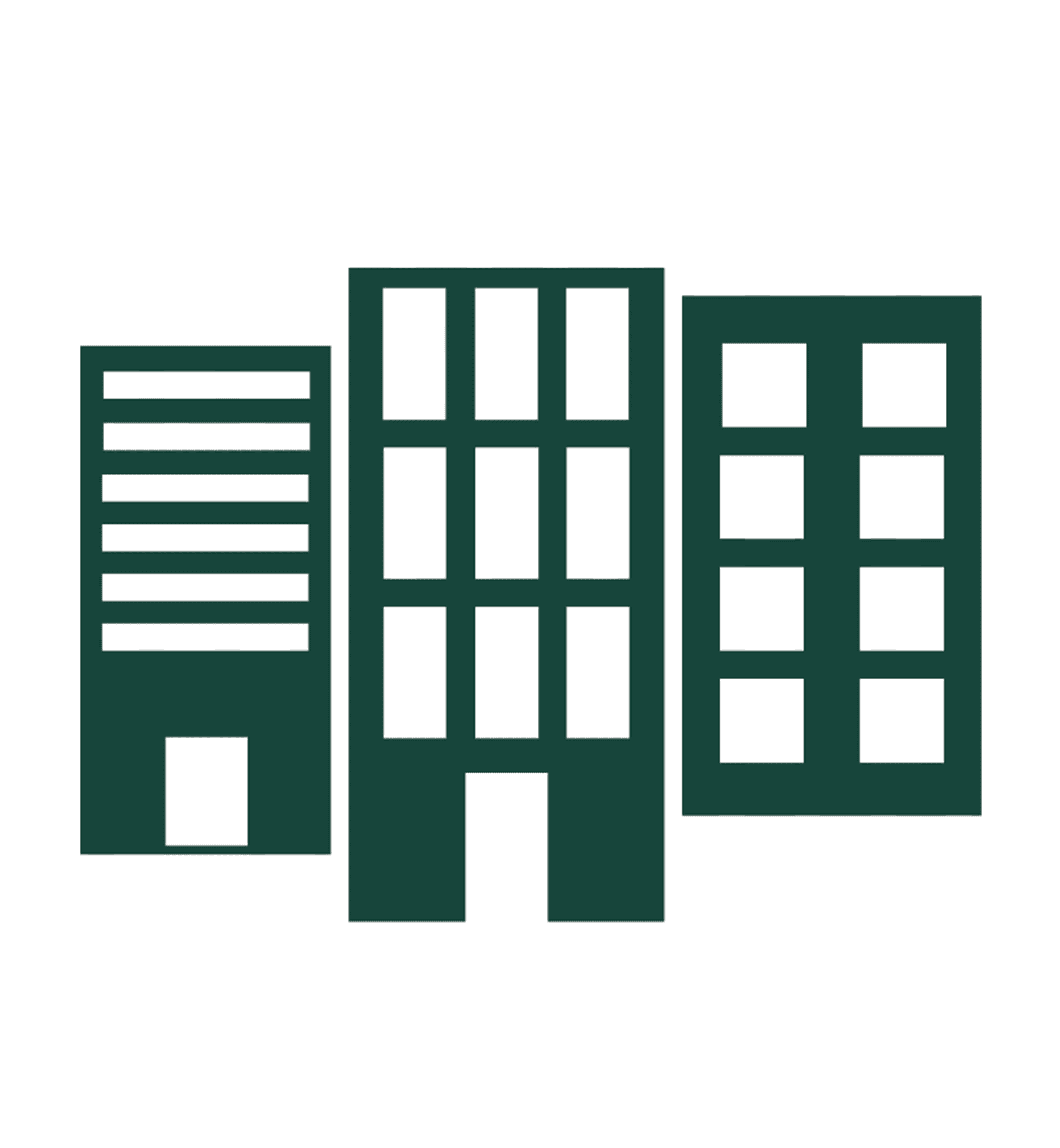
Dynamic Buildings
Smart Homes allow consumers to create a tailored ecosystem designed around their comfort and convenience. Ongoing advances at MSU help consumers customize their in-home experience for a truly unique living space.
- An assessment of opinions and perceptions of smart thermostats using aspect-based sentiment analysis of online reviews
- Occupant-Dependent Residential End Use Load Profiles for Demand Response Under High Renewable Energy Scenarios
- Safe Energy Savings Through Context-Aware Hot Water Demand Prediction
- Real-time Deep Neural Networks for internet-enabled arc-fault detection
- Activity Profiles of Occupants in Residential Buildings Using the American Time Use Survey Data
- Effective Features to Predict Residential Energy Consumption Using Machine Learning